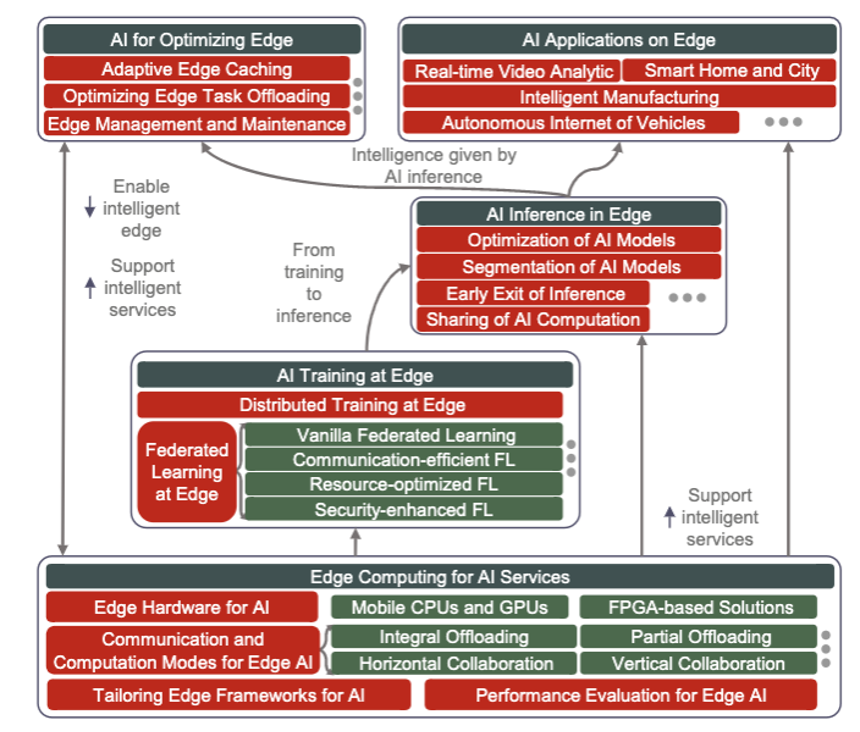
Introduction:
In the dynamic landscape of artificial intelligence (AI), the convergence with edge computing has given rise to a paradigm shift – the Intelligent Edge. This transformative fusion brings AI capabilities closer to the source of data, paving the way for more efficient, responsive, and intelligent systems. In this blog post, we will delve into the various facets of deploying artificial intelligence at the edge, exploring AI applications, inference, computing, training, and optimization in the context of edge computing architectures.
- AI Applications on Edge: Technical Frameworks for Intelligent Services:
The marriage of AI and edge computing opens up new horizons for intelligent services. Technical frameworks systematically organize the coexistence of edge computing and AI to deliver impactful applications. Whether it’s real-time analytics, image recognition, or predictive maintenance in IoT devices, the Intelligent Edge leverages frameworks that orchestrate the seamless integration of AI, ensuring that applications are not only intelligent but also tailored to the constraints and opportunities presented by edge environments.
- AI Inference in Edge: Practical Deployment for Varied Requirements:
AI inference at the edge is the backbone of practical deployment, catering to diverse requirements such as accuracy and latency. The edge computing architecture allows for localized decision-making, reducing the need for data to traverse long distances to a centralized server. This results in faster response times and is particularly crucial for applications like autonomous vehicles or industrial automation, where split-second decisions can make a significant impact.
- Edge Computing for AI: Adapting Platforms for Network, Hardware, and Software:
Adaptation is key when it comes to integrating edge computing with AI. The edge computing platform undergoes transformations in network architecture, hardware, and software to support AI computation efficiently. This adaptation ensures that the edge is not just a processing node but a smart and capable entity that contributes to the overall intelligence of the system.
- AI Training at Edge: Overcoming Resource and Privacy Constraints:
Training AI models at the edge introduces a set of challenges, notably resource and privacy constraints. Edge devices, often resource-constrained, require innovative solutions for distributed AI training. Additionally, privacy concerns mandate that training occurs locally, ensuring sensitive data remains on-premises. Overcoming these challenges is critical for empowering edge devices with the capability to learn and adapt to changing environments autonomously.
- AI for Optimizing Edge: Enhancing Edge Computing Networks with Intelligence:
The application of AI isn’t confined to edge devices alone. AI plays a pivotal role in optimizing various functions of edge computing networks or systems. From intelligent edge caching to dynamic computation offloading, AI ensures that resources are utilized efficiently, latency is minimized, and the overall performance of edge computing networks is optimized to meet the demands of real-world applications.
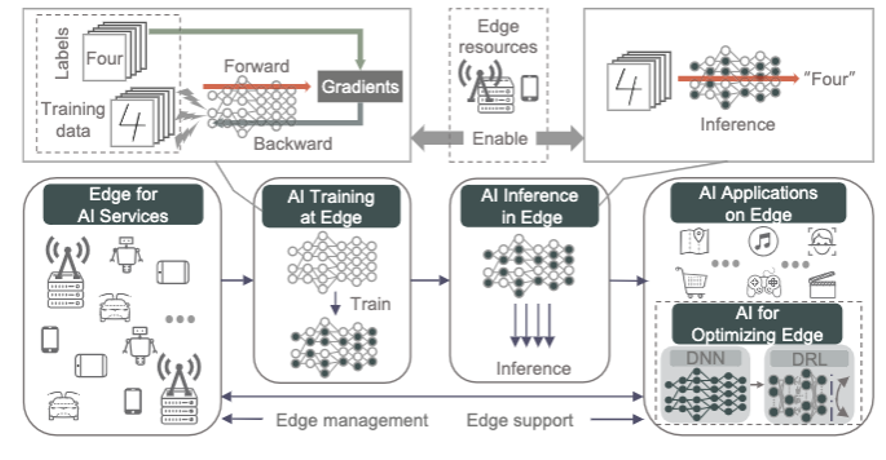
Conclusion:
In the intricate dance between AI and edge computing, the concept of the Intelligent Edge emerges as a powerful force driving innovation and efficiency. As we navigate the landscape of AI deployment at the edge, understanding the symbiotic relationship between AI applications, inference, computing, training, and optimization becomes paramount. The Intelligent Edge, with its promise of localized intelligence, responsiveness, and resource efficiency, stands at the forefront of reshaping the future of AI-powered systems across industries. Embracing this synergy is not merely a technological evolution; it’s a leap towards a more intelligent and connected world.